Using Data To Understand Complex Fluids
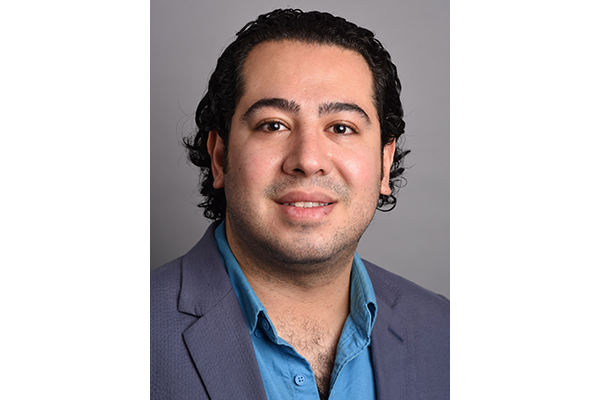
MIE Assistant Professor Safa Jamali and Postdoctoral Research Associate Anushka Jha’s research on “Data-Driven Techniques in Rheology: Developments, Challenges, and Perspective” was published in Current Opinion in Colloid & Interface Science. The research examines the different data-driven techniques in rheology, the branch of physics that focuses on the deformation and flow of matter.
Abstract:
With the rapid development and adoption of different data-driven techniques in rheology, this review aims to reflect on the advent and growth of these frameworks, survey the state-of-the-art methods relevant to rheological applications, and explore potential future directions. We classify different machine learning (ML) methodologies into data-centric and physics-informed frameworks. Data-centric methods leverage conventional ML techniques to uncover relationships within specific datasets, demonstrating success in rheological properties prediction, material characterization, properties optimization, and accelerated numerical simulations. Physics-informed machine learning combines physical laws and domain knowledge with data to produce generalizable and physically consistent predictions, proving effective in solving rheological differential equations, utilizing multi-fidelity datasets to enhance predictions, and constitutive modeling. The paper also discusses the limitations of these approaches and the ongoing efforts to address them. Looking ahead, this article emphasizes the need for explainable ML techniques to enhance transparency and trust, improved tools for uncertainty quantification. These advancements could significantly transform rheology and non-Newtonian fluid mechanics by enabling more robust, insightful, and efficient data-driven methodologies.